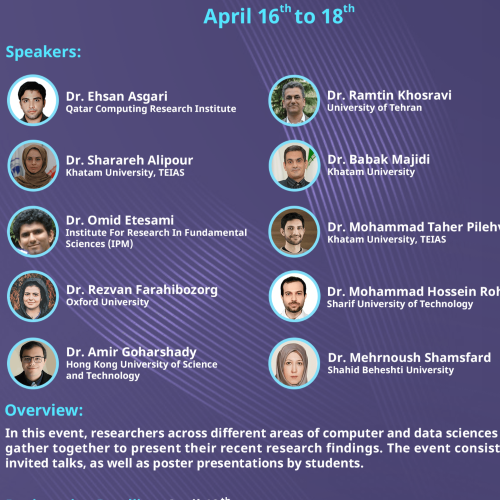
Frontiers in Computer and Data Sciences
April 16 to 18, 2024
TeIAS/Khatam computer scientist, Dr. Sharareh Alipour’s paper with her collaborators from Sharif University of Technology was accepted at the SODA 2024. SODA is among the top 3 computer science conferences. The title of this paper is “Partial coloring complex, vertex decomposability and Tverberg’s theorem with constraints”. The paper gives an application of topology to problems ranging from combinatorics to computational geometry and solves a long standing conjecture about Tverberg’s theorem with constraints.
April 16 to 18, 2024
March 05, 2024
March 6, 2024
TeIAS/Khatam computer scientist, Dr. Sharareh Alipour’s paper with her collaborators from Sharif University of Technology was accepted at the SODA 2024. SODA is among the top 3 computer science conferences. The title of this paper is “Partial coloring complex, vertex decomposability and Tverberg’s theorem with constraints”. The paper gives an application of topology to problems ranging from combinatorics to computational geometry and solves a long standing conjecture about Tverberg’s theorem with constraints.
April 16 to 18, 2024
March 05, 2024
March 6, 2024